
THE KORNGREEN LAB
Cellular and Computational Neurophysiology
DENDRITIC COMPUTATION
We apply a combination of experiment and theory to provide a biophysically realistic model for the apical dendrite of L5 pyramidal neurons in the neocortex. Within the framework of this project, we have utilized genetic algorithms for constraining compartmental models of neurons with non-homogenous distributions of ion channels (Keren et al., 2005; 2009) and voltage-gated channels (Gurkiewicz and Korngreen, 2007; Gurkiewicz et al., 2011). We have recently developed an experimental peeling procedure that greatly reduces the complexity of the numerical optimization problem (Keren et al., 2009). This procedure allowed us to provide a reduced model for the apical dendrite of L5 pyramidal neurons (Keren et al 2009). This model predicted many of the properties of the back-propagating action potential. The next stage of the project was to constrain a biophysical model for the dendritic calcium spike in these neurons. First, we have characterized the types and properties of voltage-gated calcium channels in L5 pyramidal neurons (Almog and Korngreen, 2009). Then we optimized a detailed ionic mechanism for the generation and propagation of dendritic spikes in neocortical L5 pyramidal neurons. The model predicted an ionic mechanism for many experimental results recorded from the apical dendrite of L5 pyramidal neurons, including generation of a dendritic spike. Moreover, the model validates a new methodology for mechanistic modeling of neurons in the CNS (Almog and Korngreen, 2014).
Themes
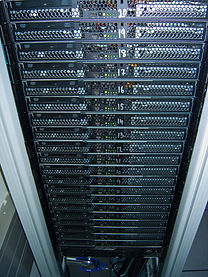
HIGH PREFORMENCE COMPUTING
A substantial computational force is required in order to perform complex computations. Over the past decade we constructed several Linux clusters, the current one containing 160 CPUs. To increase our computation power even further we started using graphic cards as computing engines. I was fortunate to receive funding and equipment donation from the graphic card company NVIDIA. We just published a manuscript detailing a new numerical code for the GPU that sped the execution of part of our optimization algorithm by man orders of magnitude (Ben-Shalom et al., 2012).


BASAL GANGLIA SYNAPTIC DYNAMICS
The basal ganglia (BG) are a group of deep brain nuclei, strongly connected with the cerebral cortex, thalamus and other brain areas, that act as a cohesive functional unit. The basal ganglia are associated with a variety of functions, including voluntary motor control, procedural learning relating to routine behaviors or "habits" such as bruxism, eye movements, and cognitive, emotional functions. Many neurons in the BG display highly dynamic and rapid action potential firing. From my point of view, this makes them an interesting system in which to investigate the contribution of synaptic integration on network activity. We are collaborating with Prof. Bar-Gad and we are utilizing the similarities between the primate and rodent systems in our labs to try and bridge the gap between the integration at the cellular level to network function. Several joint manuscripts resulted from this close collaboration and we currently share one grant on this topic as well.
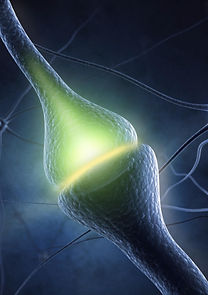
SYNAPTIC PLASTICITY AND POSTSYNAPTIC DYNAMICS
Synaptic plasticity is an important part block of our ability to learn and remember. In the recent years we have investigated the link between synaptic plasticity and neuronal dynamics using both experiment and theory. We have shown that high and low frequency stimulation of the subthalamic nucleus induce prolonged changes in subthalamic and globus pallidus neurons (Lavian et al., 2013). This result may be of importance to the design of deep brain stimulation (DBS) protocols that may be applied for treating the symptoms of Parkinson’s disease. Based on these and other studies we were able to simulate the impact of short-term synaptic plasticity on network activity during repetitive stimulation (Brody et al 2013). We are currently investigating the short and long term plasticity of additional microcircuits in the CNS. We are especially interested in the impact of these changes on the computational properties of the single cell and the small neuronal network.